Quick Demo Video for Check-it-Out
General Music & Electronic Dance Music (EDM)
String & Orchestra
Piano
Guitar
Saxophone
MusicLDM Generation from Rilke's Poem
We made a creative process among MusicLDM, ChatGPT, and Rilk's Poem "End of Autumn": we first discuss this poem with ChatGPT and let it generate desscriptions of each poem line. Then, we send these descriptions to MusicLDM and generate differnet pieces of music samples. Finally, we combine these samples together to get the generation of MusicLDM, from Rilk's Poem "End of Autumn". Such creative process gives a potential application of how text-to-music generation systems can establish a new paradigm of machine composition, by leverging the advanced techniques of diffusion models and large language models, to benefit the computer music area.
Similarity on Training Set
In order to verify if an audio track in the training set is copied by the model. We use the CLAP score to measure similarity between each audio embedding in the training set, to its most similar audio embedding from the generation pieces. Therefore, we can verify if the model could leverage Beat-Synchronous Latent Mixup (BLM) to prevent copying the audio tracks in the training set when performing the generation.
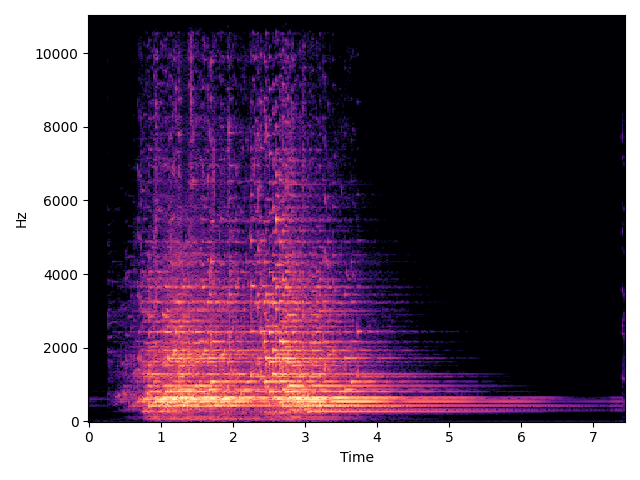
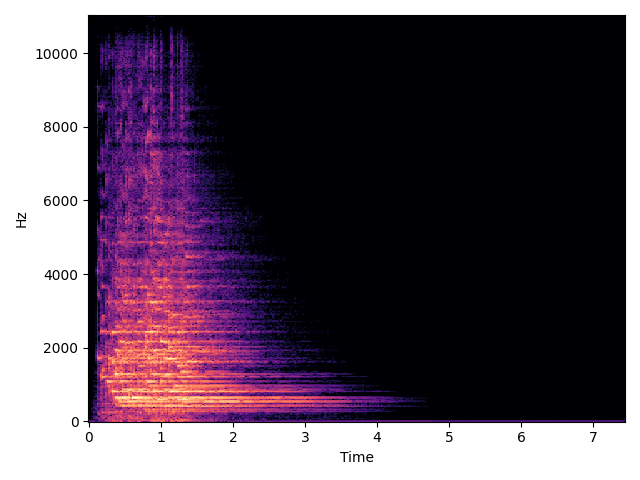
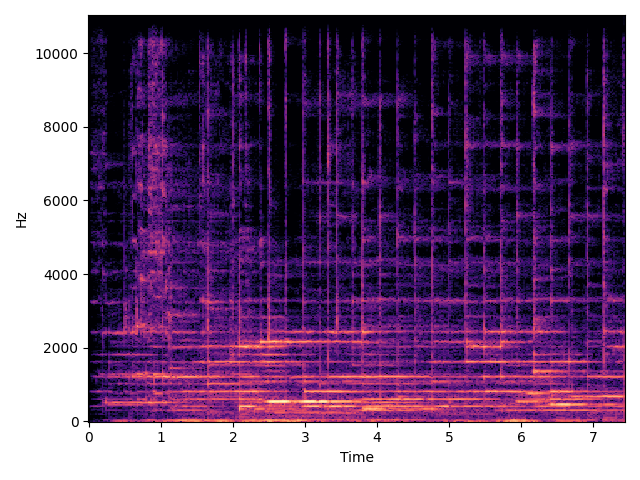
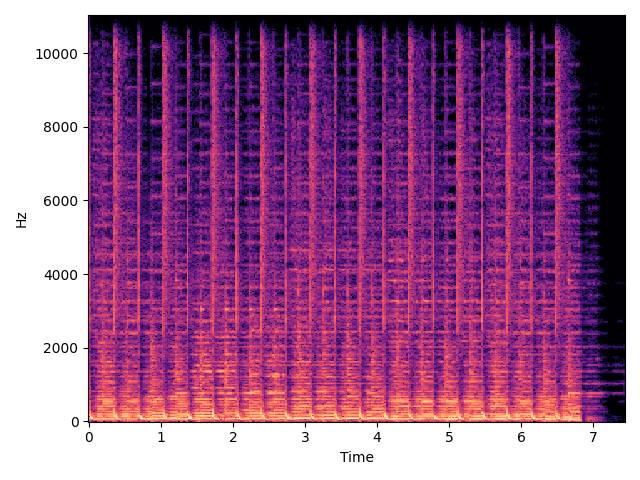
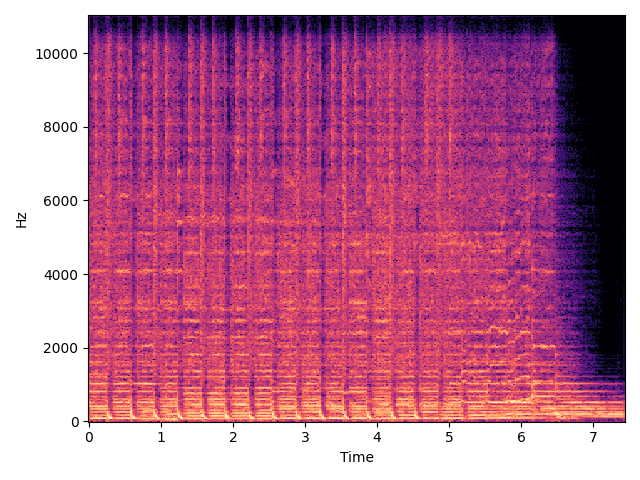
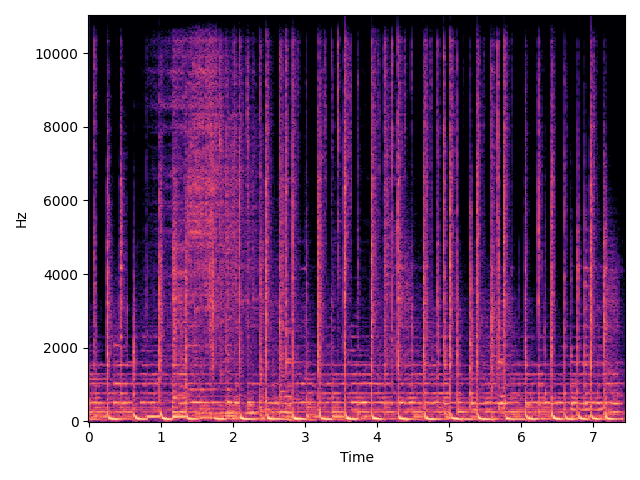
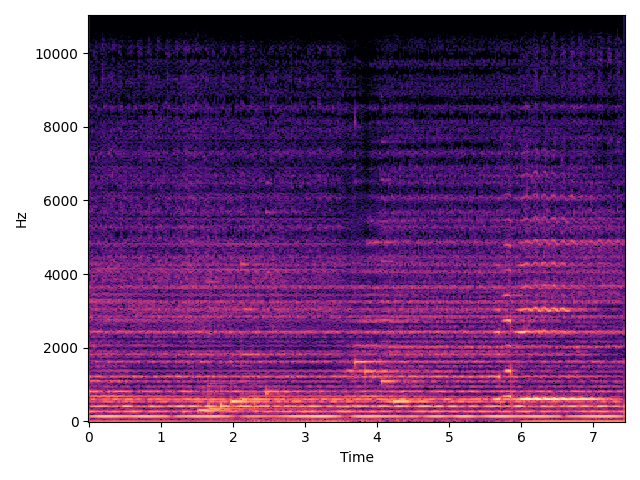
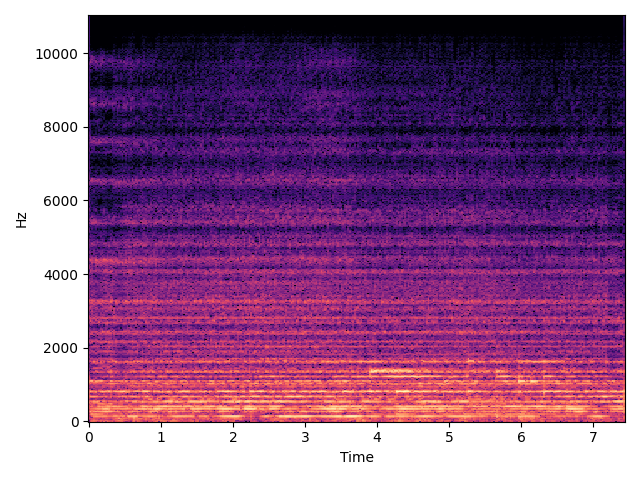
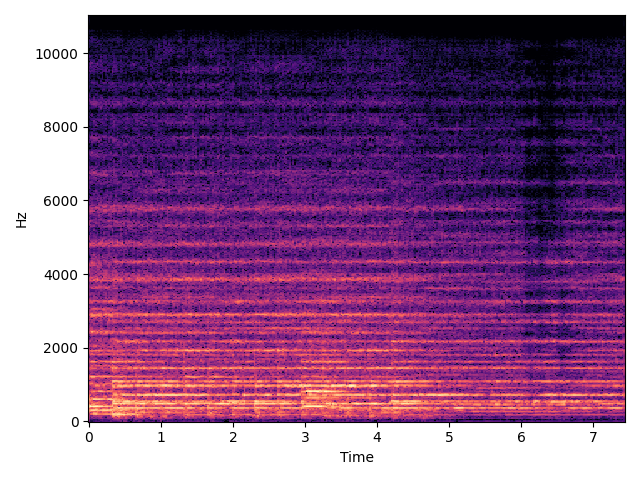
Similarity on Test Set
Similarily and Reversely, we use the CLAP score to measure similarity between each audio embedding from the generation pieces, to its most similar audio embedding in the training set. This is the verification, in terms of the recall, to detemine if BLM can prevent the model from copying the audio tracks in the training set.
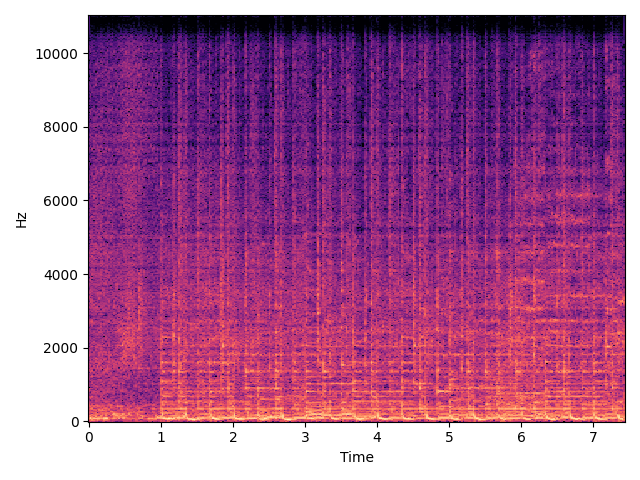
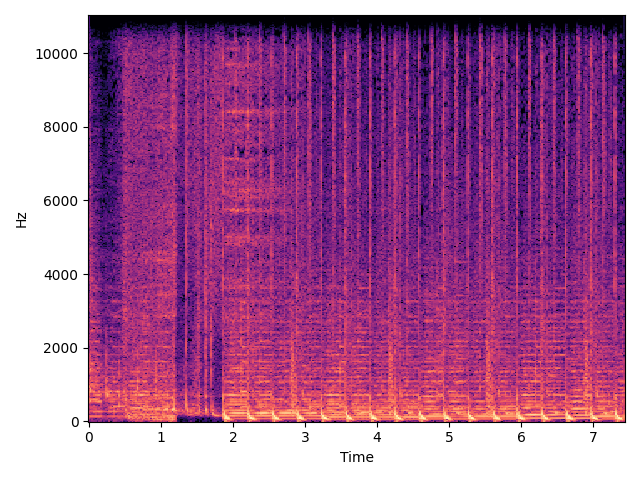
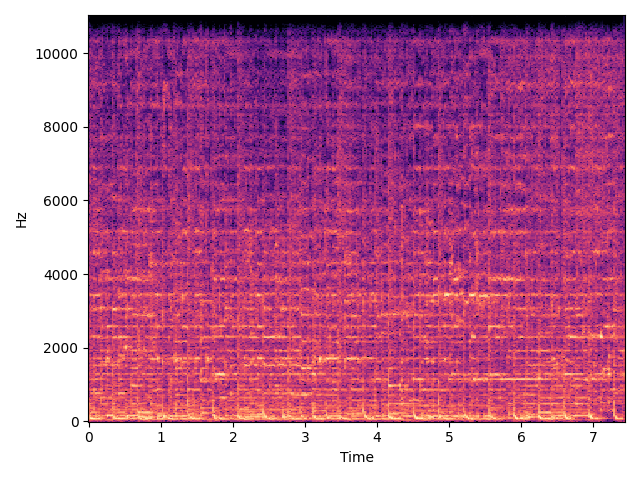
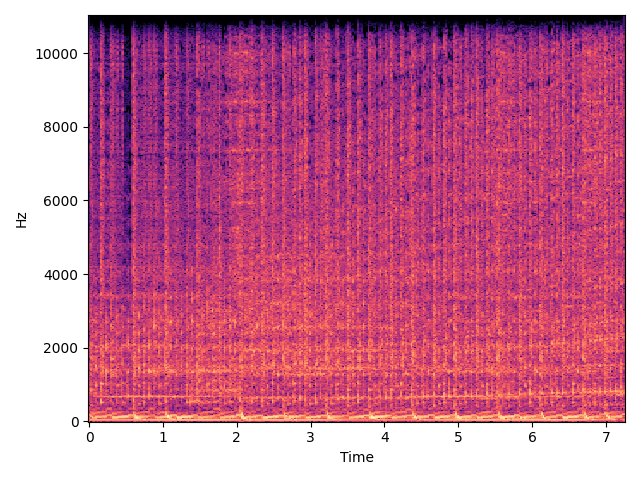
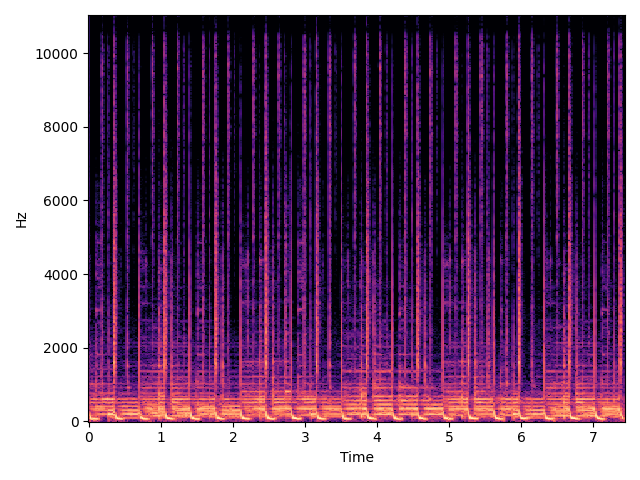
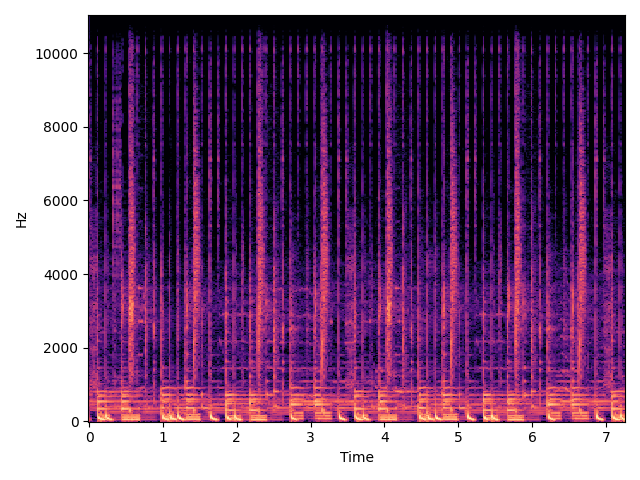
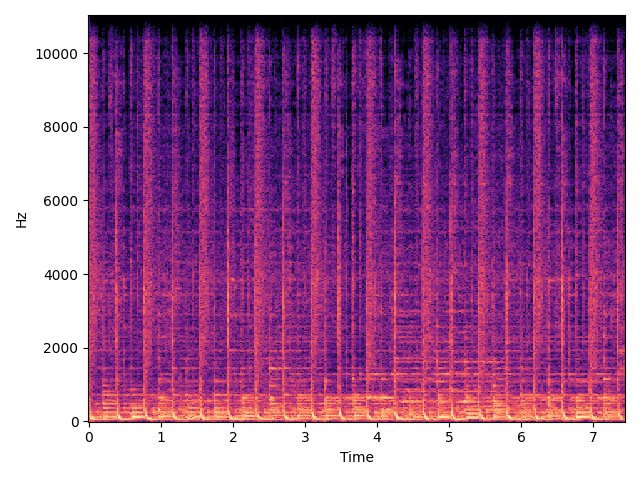
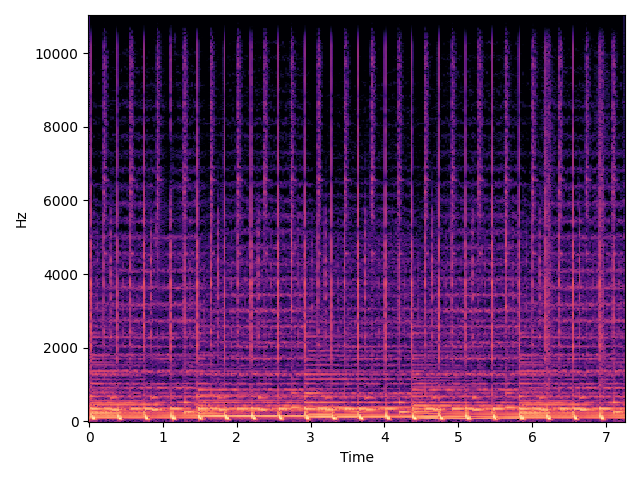
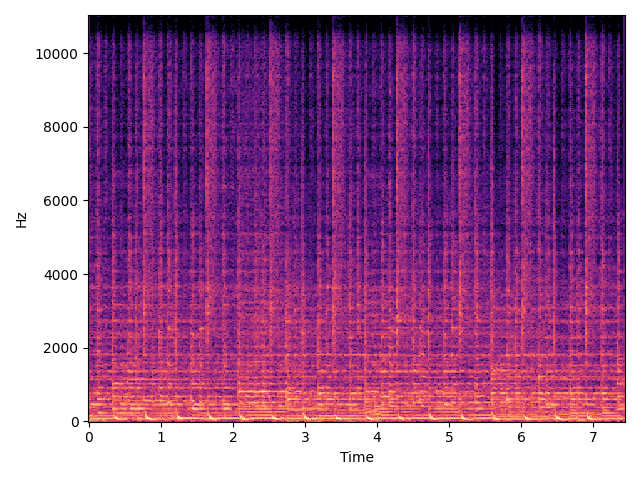
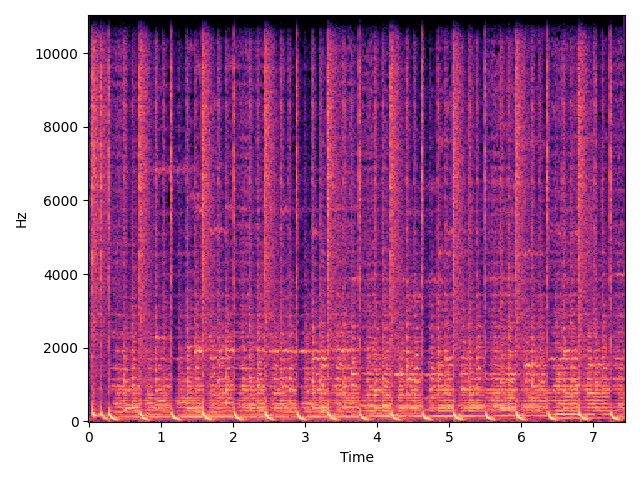
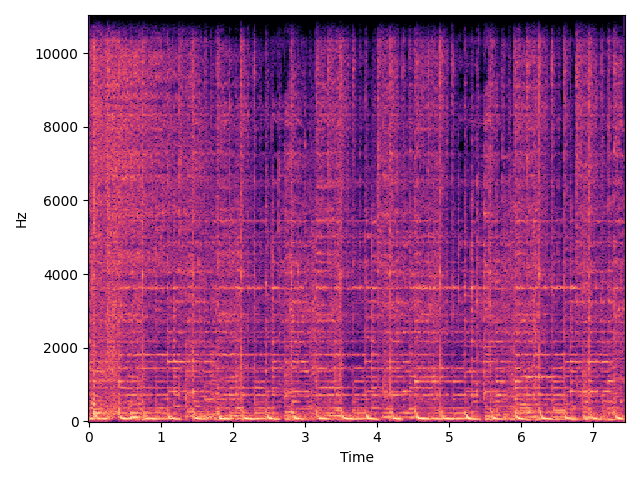
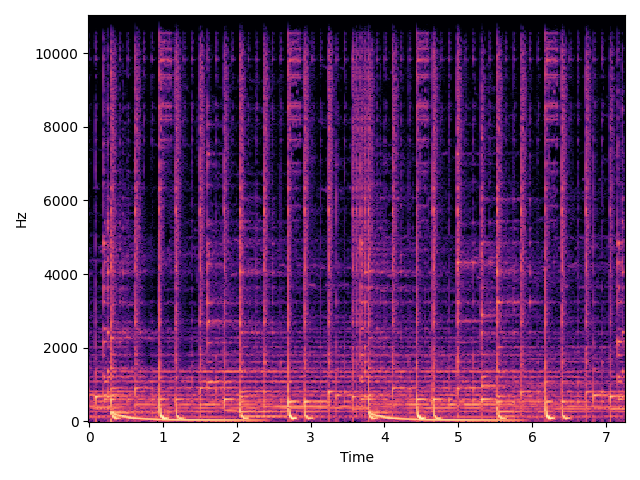